1. First, what does insurance fraud entail?
For many years, insurance fraud has been an ongoing issue that costs businesses billions of dollars annually and raises premiums for law-abiding policyholders. The conventional techniques of identifying fraudulent claims, such as manual audits or basic rule based systems, frequently prove insufficient when fraud schemes get more intricate and pervasive. Machine learning (ML) is useful in this situation. Insurance companies can save time, money, and resources by detecting fraud more accurately and efficiently by utilizing ML algorithms.
But how precisely does machine learning assist in the identification of insurance fraud? Let’s investigate more closely.
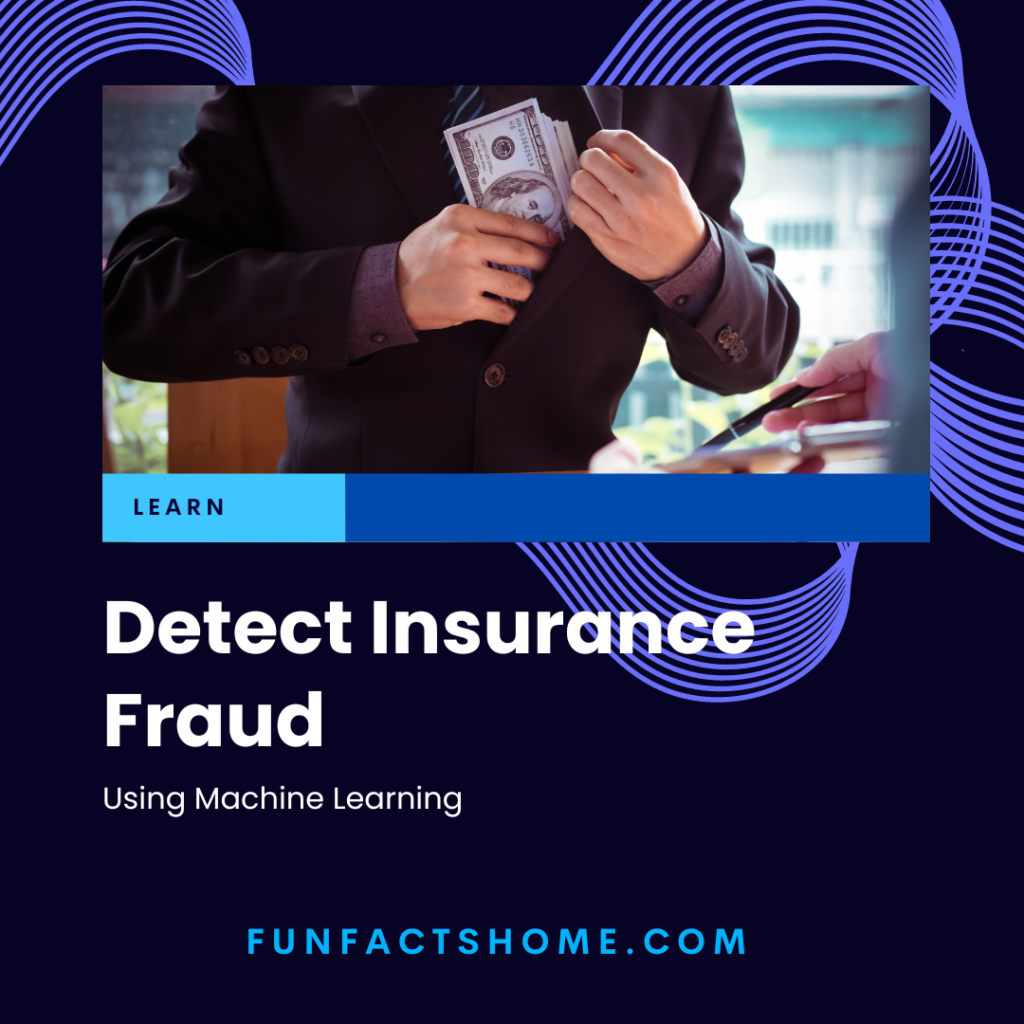
When someone intentionally files a fictitious or inflated insurance claim in order to receive unjustified compensation, it is known as insurance fraud. It can appear in various ways, such as: Inflated medical claims Staged auto accidents Falsified property damage reports Fake death certificates in life insurance
Policyholders, claimants, service providers (such as physicians or repair shops), and even insurance employees are all capable of committing fraud. Insurance companies may find it challenging to manually examine every claim in detail due to the volume of them, which allows fraudulent claims to evade scrutiny. This is where the enormous dataset processing and analysis capabilities of machine learning come in handy.
2. Fraud Detection Through Machine Learning
Computers can now identify patterns in past data and use that knowledge to inform decisions and forecasts thanks to machine learning. When used to detect insurance fraud, machine learning models examine sizable datasets of previous claims, both fraudulent and legitimate, in order to spot any patterns or unusuality’s that might point to fraud. This is how it operates;
Data Collection: For machine learning models to function well, a lot of data is required. This can include customer profiles, claim details, policy information, payment histories, and any past fraud instances in the insurance industry. After that, the data is cleaned and ready for the model, guaranteeing its accuracy and errorfree nature.
Training the Model: Machine learning models get their pattern recognition skills from this data. For instance, the model might discover that inflated medical claims typically have particular types of documentation errors, or that fraudulent auto claims frequently entail repairs at particular pricey body shops. Future claims can then be marked as possibly fraudulent based on these patterns.
Anomaly Detection: With proper training, machine learning models are able to identify anomalies, or claims that don’t fit the usual pattern. The system may flag cases for additional investigation, for example, if an individual files an abnormally high number of claims in a short amount of time or if a repair cost is disproportionately higher than the average for claims of a similar nature.
3. In Fraud Detection, supervised vs. Unsupervised Learning
In fraud detection, supervised learning and unsupervised learning are the two primary forms of machine learning.
Supervised Learning: This method uses a labeled dataset to train the model, with claims clearly classified as fraudulent or not. The model gains an understanding of fraud through supervised learning by using historical examples. After training, the model can score new claims according to how likely they are to be fraudulent by using what it has learned. Frequently employed in supervised learning are algorithms such as support vector machines (SVM), random forests, and decision trees.
Unsupervised Learning: Unsupervised learning aids in the identification of anomalous patterns that depart from the norm in situations where there are no labeled instances of fraud. Clustering algorithms, for example, can cluster claims with similar features, which facilitates the identification of outliers. These anomalies might point to previously undiscovered forms of fraud. Unsupervised learning is especially helpful in detecting new fraud schemes that lack a well-established historical precedent.
4. Most Important Advantages of Machine Learning for Fraud Detection
Compared to conventional fraud detection techniques, machine learning provides the following benefits:
Speed and Efficiency: ML algorithms are able to quickly identify suspicious claims by processing large volumes of data in real time. Insurance firms are now able to react to possible fraud more quickly than they could have with manual audits.
Enhanced Accuracy: Machine learning can identify nuances in patterns and correlations that simple rule-based systems or humans might miss. As a result, there are fewer false positives regular claims that are mistakenly reported as fraudulent—and actual fraud is more precisely identified.
Scalability: Machine learning models can expand to handle larger datasets without sacrificing accuracy or speed as insurance companies expand and the volume of claims rises. This is particularly helpful in sectors with high claim volumes, such as health and auto insurance.
Adaptability: As scammers come up with new ways to take advantage of the system, fraud schemes change over time. Retraining machine learning models on a regular basis can help them adjust to these shifts and maintain the effectiveness of the detection system.
5. Difficulties and Things to Think About
Even though machine learning has a lot of benefits for fraud detection, it’s not a perfect answer. Insurance companies face various challenges that they must take into account.
Data Quality: The quality of the data used to train machine learning models determines its performance. The model’s predictions will suffer if the data is biased, erroneous, or incomplete. Having high-quality data is necessary to detect fraud effectively.
Interpretability: It can be challenging to understand some machine learning models, particularly the more intricate ones like deep learning. This “black box” problem makes it difficult for insurers to explain decisions to customers or regulators because it makes it difficult to understand why a specific claim was flagged as suspicious.
False Positives: Although machine learning increases accuracy, there is always a chance of incorrectly classifying legitimate claims as fraudulent. Insurers must find a way to minimize inconvenience to truthful clients while simultaneously identifying actual fraud.
6. Machine Learning’s Practical Uses in Insurance Fraud
With impressive results, many insurance companies are already using machine learning to detect fraud. As an illustration:
Allstate Insurance analyzes auto claims using machine learning algorithms and looks for unusual trends in vehicle damage reports.
Progressive Insurance has put in place an MLbased system that examines claims for irregularities based on a number of variables, including the date, location, and claimant history.
Zurich Insurance specializes in property and casualty insurance claims; utilizing AI and ML, the company sifts through massive datasets to identify fraudulent activity in real time.
7. Fraud Detection’s Future
It is likely that machine learning will be even more integrated with cutting
edge technologies like blockchain and artificial intelligence (AI) in the
future of insurance fraud detection. A blockchain could offer an unchangeable
record of transactions, making it more difficult for data manipulators to
commit fraud. AI, on the other hand, can be used to aggregate data from various
sources, including social media usage, telematics (used in auto insurance), and
Internet of Things devices, to produce a more comprehensive picture of a
claimant’s actions.
In the end, machine learning will remain crucial in assisting insurers in
staying one step ahead of fraudsters, safeguarding their financial interests,
and guaranteeing more equitable premiums for clients.
In summary:
Insurance companies are detecting and preventing fraud in a whole new way thanks to machine learning. Compared to conventional methods, machine learning (ML) provides a more accurate, efficient, and scalable solution by analyzing large amounts of data, learning from patterns, and continuously adapting to new fraud schemes. While there are certain issues to take into account, like the interpretability of models and the quality of the data, machine learning has many advantages when it comes to identifying insurance fraud. Technology will become an even more potent weapon in the fight against fraud as it develops further, guaranteeing a more safe and open insurance market for everybody.